(SMT Data Challenge Finalist paper)
Introduction
With the advent of player and ball tracking data to the game of baseball, we are able to answer, in a statistical and precise fashion, questions that have been left to human feel for centuries. One facet of the game, outfielder coverage, is the focus of this analysis. When the ball is hit in the air toward an outfielder, their strategy is simple and almost perpetually consistent: catch the ball to record an out. The fielder is almost always inclined to try his hardest to catch the ball, regardless of the number of outs, what men are on base, the inning. The unvarying nature of this goal makes fly ball tracking analysis ripe for statistical modeling.
Before tracking data, evaluating outfielder coverage was a challenge that failed to capture the whole story due to the lack of context. Somewhat savvy analysts used metrics like “fielding percentage,” which attempts to capture a player’s consistency by dividing their putouts (outs recorded with a catch) and assists (outs recorded with a throw) by their putouts, assists, and errors (super arbitrary mark of a substantial mistake). At the high level, this metric tries to capture the proportion of opportunities that are successful, similar to how batting average works for hitters or how free throw percentage works in the NBA. However, there are many contextual blindspots to this method and other non-tracking outfield metrics:
A success is deemed a success, no matter how improbable. All outfield catches are labeled as putouts, without knowing how difficult the play actually was. A “can of corn” (extremely easy pop out) is valued the same as a “web gem” (spectacular play).
Incompetence is excused. Imagine a scenario where a fielder is slow to react to a ball headed his direction, reads the flight path poorly, and the ball lands in front of him on his side of the gap for a single. To the naked eye this seems like a ball our fielder should have caught, but as humans we have an extremely rough and biased sense of how likely an outfielder can make a catch in that scenario. A scout may write that he “lacks anticipation” but that conclusion lacks objectivity and fails to answer how much and how consistently he is lacking. Since this play is not recorded as a putout, assist, or error, the slow-reacting outfielder’s fielding percentage is unimpacted.
Errors are too subjective. Check out this play from July 2022: https://baseballsavant.mlb.com/sporty-videos?playId=558f79ac-b360-4d95-a037-f9b80c68574f After nearly six seconds in the air, the ball lands about 20 feet behind where center fielder Jarren Duran started the play. Based on these parameters, MLB tracking’s Statcast gave Duran a 99% chance of making this play, which explains Raimel Tapia’s obvious displeasure upon contact. Duran proceeds to lose the ball in the lights as he takes a couple steps forward, completely unaware that the ball is traveling over his head, allowing Tapia to sprint around the bases scoring four runs. Despite the precedent that MLB outfielders are successful on similar plays 99% of the time, Duran is not charged with an error by the official scorer, thanks to its entirely subjective and inconsistent nature. This massive blunder does not show up against him in his fielding percentage.
This analysis breaks free of antiquated fielder evaluation techniques by assigning an objective catch probability to each outfielder opportunity. Players that exceed their expectations are deemed more successful at fly ball tracking than their counterparts. This process is useful for any team that wishes to more accurately evaluate outfielders, with or without the benefits of tracking data.
Data Preparation
The anonymized minor league baseball data given for this competition involves 97 games from two different organizations who hosted their opponents for short series. Organization A has three levels: 1, 2, and 3 (think AAA, AA, A) with 16, 18, and 27 games played respectively over three seasons. Organization B has one level with 36 games played over four years. The nature of the data is such that game events (player hits a double, runner advances on wild pitch, etc.) must be more or less inferred. We are without play-by-play data with tags like “Raimel Tapia hits an inside-the-park grand slam on a fly ball to center field.” For a given game scenario, it’s difficult to say for certain how many outs have been recorded or even what the score of the game is without manual intervention.
This obscurity is consistent with fly ball data where we are not informed if a player caught the ball on the fly, only that the ball was “acquired” by a particular player. For plays where the ball was batted in to play, a successful catch could, in theory, be classified as the ball being acquired by a player before any bounce, or deflection is recorded, but because bounces are manually encoded, unlike automatically generated data like player and ball coordinates, this data is extremely inconsistent. The inconsistencies were found at the Team B stadium, where the maximum amount of bounces in one year could be the near minimum in another year (see image). Six games had less than 10 recorded bounces which is obviously flawed.
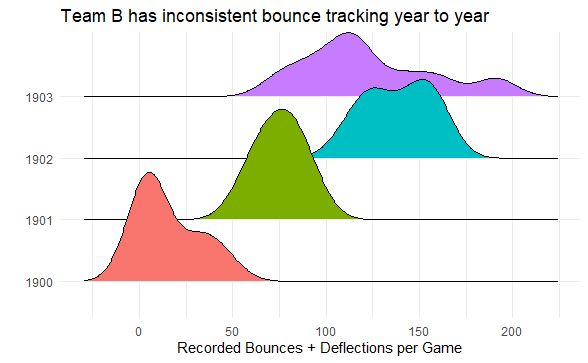
Below is an example play from a Team B game where the recorded contact point (green line) and acquire point (red line) are plausible based on the height of the ball (y-axis). Unfortunately, no events were recorded in the time between contact and retrieval, even though it is apparent based on the z-axis (height) of the baseball, the ball bounces at least twice. Plays like these at Team B’s stadium threw a wrench at any attempt at classifying batted balls without a recorded bounce prior to possession as successful catches.
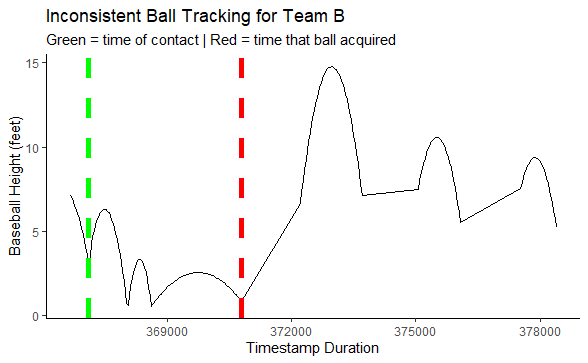
Fortunately, Organization A’s bounce tracking is statistically consistent year to year and across levels of play. Because we have much more faith in the plausibility of their data, this analysis will focus solely on the 61 games played at their stadium. This gives us advantages like having a controlled ballpark, and the ability to make more apt comparisons of players’ ability both across the organization and among opponents.
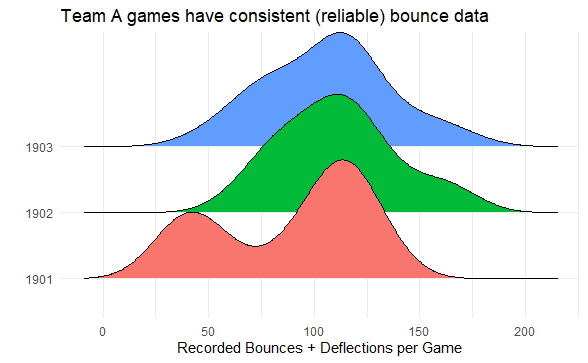
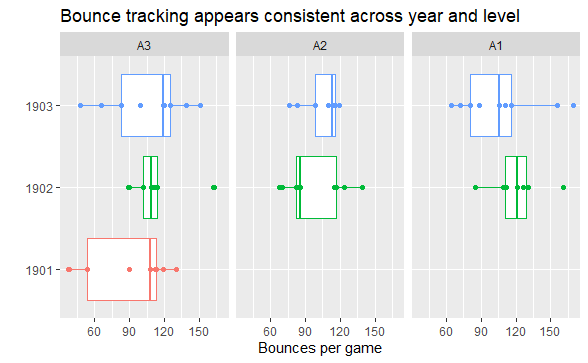
Predictions
After the data is properly filtered and features are added (see Appendix), a relatively simple and extremely effective model trained on Organization A’s 1901-1902 seasons is used to make catch probability estimates for each outfielder opportunity in the 1903 season. To make an estimate on how likely a given fly ball to the outfield is to be caught, the model takes into account three factors:
Duration: The time difference between the pitch and when the ball reaches its landing (or catching) point. Fly balls with more hang time allow the outfielder to cover a larger area, making them more likely to be caught (see chart). Balls in the air less than three seconds are borderline uncatchable, while pop flys with longer than six seconds from pitch to catch are almost always caught. These opportunities are assigned extremely low and high catch probabilities, so when a player does what is expected of them, we learn almost nothing about their true outfield skill.
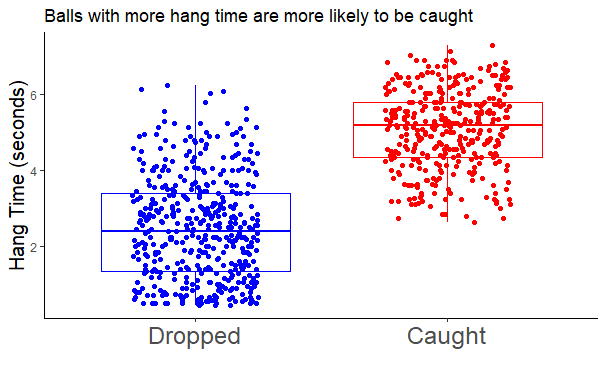
Distance: The straight line distance from the outfielder at the pitch to where the ball eventually lands or is caught. Unlike duration, distance has a negative effect on catch probability. The more area an outfielder must cover, the less likely he is to make a successful catch before the ball hits the field (see chart). Because distances greater than 100 feet are nearly impossible to cover in time and not be successfully caught by another outfielder, players in these situations are penalized virtually nothing when they fail. Distance and duration by themselves are not quite enough to capture catch probability sufficiently, but together they paint an extremely strong picture. For example, a handful of pop-ups with a duration of six seconds (see above) are not caught, presumably because of their great distance required.
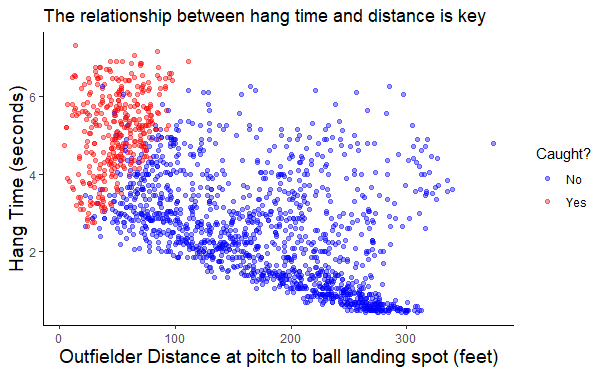
Direction: Whether or not the outfielder must travel back (away from home plate) to reach the ball. Perhaps a bit less intuitive, durations and distances are not all created equal; fly balls where a player must travel backwards adds increased difficulty. These plays usually require the player to sprint while looking over the shoulder, slowing them down, and to gauge the outfield wall’s location in order to ensure their own safety. The model found that this knowledge of the catching/landing spot’s orientation to where the fielder began improves catch predictions. At a constant duration and distance, a player making a successful catch on a ball hit behind him, away from home plate, will be rewarded more than his counterparts. The non-uniformity in catch difficulty at the same distance presents an interesting opportunity for teams strategizing against a hitter. It is not sufficient to place an outfielder at the center of where a batter may hit the ball but rather behind that mark, to account for the added difficulty of traveling backwards.
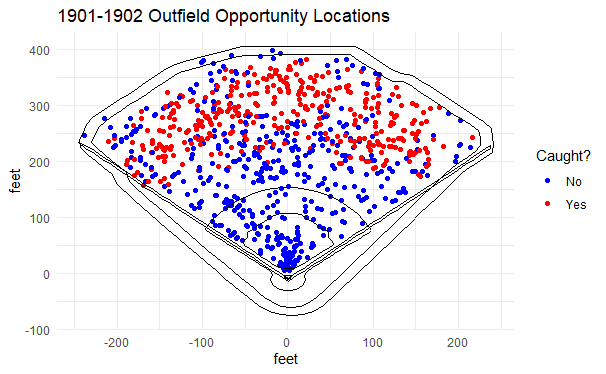
Results
Model Effectiveness
Using only these three features, the chosen model successfully classified 97% of outfielder opportunities in the 1903 season. Of balls that were actually caught, 92% were predicted to be caught (catch probability exceeding 0.5). Unsuccessful opportunities were correctly classified (catch probability less than 0.5) 99% of the time. The presented image is a confusion matrix, a graphical version of the aforementioned statistics, where values of 0 and 1 represent catch failures and successes respectively. The “misclassification” boxes (bottom left and top tight) are miniscule compared to the amount of correct classifications.
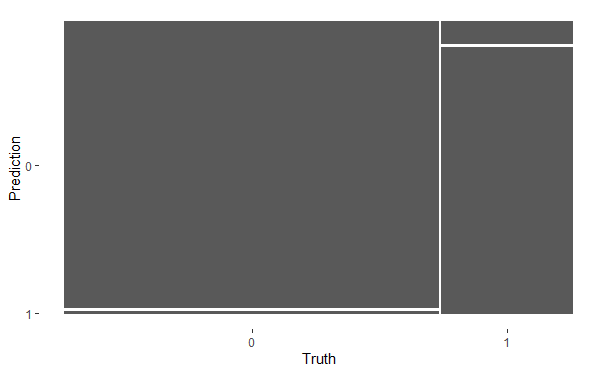
To the right is a visualization of how the model assigned catch probabilities for the 1903 season’s data, with each dot representing an opportunity. The “green belt,” plays in the area of 50 feet in 3 seconds to 100 feet in 5 seconds, are opportunities with a near coin flip catch probability. Players who consistently make these catches “on the margins” are considered more effective at fly ball coverage.
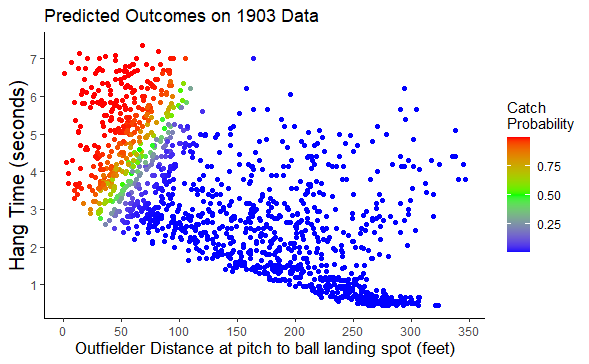
With the above chart in mind, we can achieve a better sense of our model’s strength, beyond the accuracy metric. Looking at the graph to the left, we see that nearly all of the misclassifications (red dots) are along the 0.5 catch probability line, indicating they were incorrectly guessed by a trivial amount. This is more telling of an effective model than if it were extremely confident in plays that are routinely misclassified.
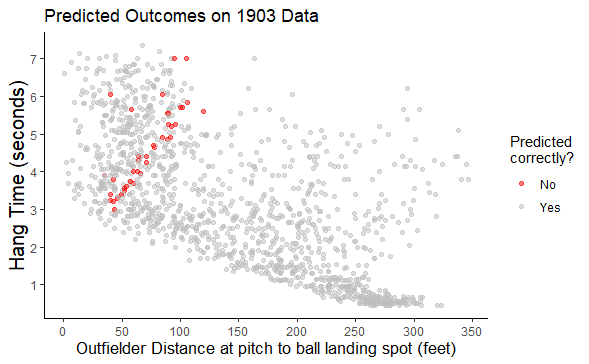
Player Evaluation
Each outfield opportunity has an assigned catch probability generated by the model and the “real-life” outcome given in the data. By comparing and aggregating these values, an “Outs Above Average” metric can be generated. Let’s say on a given play, outfielder Geoff makes a catch that has a 60% chance of success based on his distance, the hang time, and direction he must travel. The difference in the outcome and predicted success gives us his Outs Above Average (OAA) recording. We assign the catch as a 1, convert his 60% chance to a probability, and calculate 1-0.6=(+0.4) OAA. He is rewarded 0.4 outs more than an average outfielder for his efforts. If he failed to make the catch, the calculation becomes 0-0.6=(-0.6) OAA. Because we thought he would catch the ball, he is penalized more for a failure than he stands to gain on a success. Summing each of his opportunities over the entire season yields his season-long OAA. In the long run, an “average” outfield ball tracker would have his positive and negative plays cancel out equally, giving him an OAA of 0.
Here are the five best and five worst outfielders in 1903 by season-long OAA:
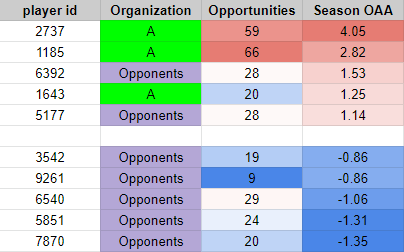
Player 2737, a center fielder on Team A3, made the most of his 59 opportunities by scoring over 4 cumulative Outs Above Average. He accounts for two of the ten most improbable catches of the season, a 22% catch where he traveled 90 feet backwards in 5.25 seconds and a 28% catch traveling 59 feet in 3.7 seconds. These two plays alone account for +1.5 OAA. In the chart to the right, each outfielder who played a game at Team A’s stadium in 1903 is identified as a dot. Most players have expected outs (the sum of their individual catch probabilities) very similar to their actual successes, indicating that there was not enough of an opportunity (each A organization team played 9 games and opponents played less than 4) for them to display differentiated fly ball skills. Teams gain very little on fly ball skills in a small sample of games with the exception of exceptional fielders like Player 2737 and 1185. A larger sample could show that these skills are differentiated in the long run, or that fly ball skills are essentially negligible.
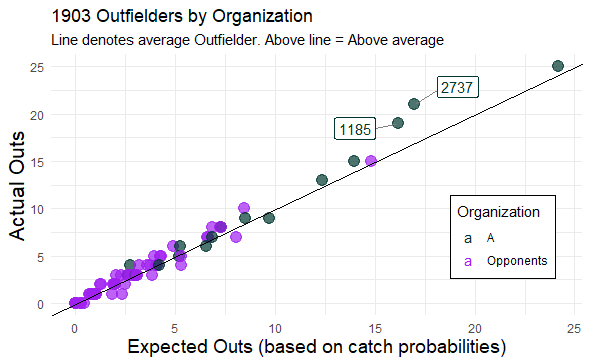
Team Evaluation
Aggregating plays by organization gives us team-level metrics to study (see table). It appears Organization A’s outfielders have superior ball tracking skills than their opponents, earning 11.5 OAA in 27 games in 1903 compared to 7.7 for their opponents (more on why these numbers fail to cancel out in the next section). Controlling for the number of opportunities is important in order to make comparisons on a more level scale. Per opportunity, Organization A added 0.019 fly ball outs vs. their opponents’ 0.011 against them.

Examining the A organization at the team level tells a bit of a different story. The vast majority of the organization’s fly ball skill is thanks to their top level team, Team A1, which has a cumulative OAA of 9.3 (see chart below). They displayed an ability far superior to their opponents. Team A2 and A3 on the other hand, were both outperformed by their opponents in OAA per opportunity. While the A organization was overall better than their opponents, the fly ball talent appears to be topheavy.
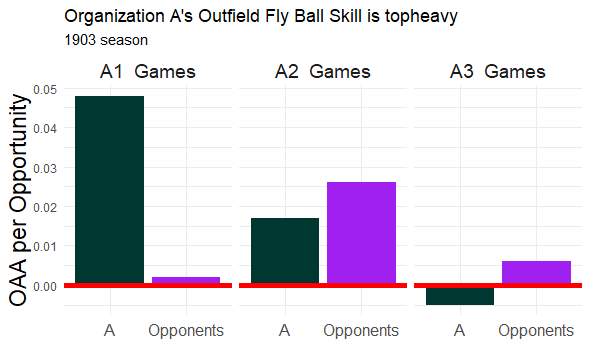
Blindspots, Uses, & Misuses
While the framework of this project is certainly applicable to MLB organizations with access to tracking data, and useful for lower-level coaches looking to adjust their intuitions, a discussion of how this project should not be used is just as important.
This framework evaluates outfielder skill on fly balls only, which is useful but is not the complete picture. In order to achieve a more all-encompassing defensive metric for outfielder ability, arm talent should be used in conjunction and run value should be factored in.
With the data given (lacking game score and outs), this topic seems reliable because outfielders generally are always inclined to try their best to make a catch, regardless of game score and outs, meaning that the lack of that data would not impact the analysis. It is not always the case that an outfielder should give everything they have to catch a ball. Injury considerations, and the risk management of failure upon diving for a ball should also play into defensive strategy.
While the model framework is valid (this same analysis could be used on MLB data just fine), the Results section is not the most accurate it could be due to the data it is trained on. First of all, data used in the model training process should be randomly distributed among all teams for less biased results rather than heavily favoring a few teams (A1, A2, and A3). For example if I trained an infielder model using only Ozzie Smith’s data, nearly every player ever would look below average. Additionally, it’s quite peculiar that Organization A and their opponents were both so far above average in the 1903 season, despite all three levels playing 9 games each. While it’s possible that they improved over the 1901-1902 seasons the data was trained on, the failure to cancel out is more likely due to bias in the training data. 53% of games in the training data involve Team A3, who we have reason to believe is the lowest level team, implying that the data is trained on generally worse players who are probably worse fielders. The bar is set relatively low, rather than the true “average,” allowing outfielders at higher levels to more easily overachieve. This could have been approached by adjusting OAA by level, but at the risk of training on too few data to make strong predictions. The OAA metrics should not be fully trusted as absolutes but they do have merit as comparison tools. The usage and tweaks of the OAA metric generated are subject to the task requested.
Appendix: Methods
Methods included in the full paper here: https://github.com/jackweyer/UCSAS-Data-Challenge/blob/main/UCSAS%20final%20paper.pdf
Comments